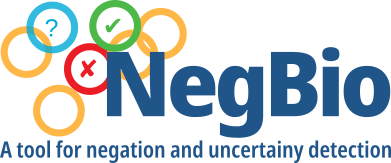
NegBio is a high-performance NLP tool for negation and uncertainty detection in clinical texts (e.g. radiology reports).
Get started
Install NegBio
Installing from source (recommended)
$ git clone https://github.com/ncbi-nlp/NegBio.git $ cd /path/to/negbio $ python setup.py install --user $ export PATH=~/.local/bin:$PATH
Installing from pip
$ pip install negbio
Prepare the dataset
The inputs can be in either plain text or BioC format.
If the reports are in plain text, each report needs to be in a single file.
Some examples can be found in the examples
folder.
Run the script
There are two ways to run the pipeline.
NOTE: If you want to process a lot of reports (e.g., > 1000), it is recommended to run the pipeline step-by-step. See User guide.
Using the CheXpert algorithm
If you want to use the CheXpert method, run one of the following lines
$ main_chexpert text --output=examples examples/00000086.txt examples/00019248.txt
$ main_chexpert bioc --output=examples examples/1.xml
Using MetaMap
If you want to use MetaMap, run the following command by replacing <METAMAP_BINARY>
with the actual ABSOLUTE
path, such as META_MAP_HOME/bin/metamap16
$ main_mm text --metamap=<METAMAP_BINARY> --output=examples examples/00000086.txt \
examples/00019248.txt
$ main_mm bioc --metamap=<METAMAP_BINARY> --output=examples examples/1.xml
Documentation
negbio documentation is available on Read The Docs.
See Getting Started for installation and basic information. To contribute to negbio, read our contribution guide.
Citing NegBio
If you're running the NegBio pipeline, please cite:
- Peng Y, Wang X, Lu L, Bagheri M, Summers RM, Lu Z. NegBio: a high-performance tool for negation and uncertainty detection in radiology reports. AMIA 2018 Informatics Summit. 2018.
- Wang X, Peng Y, Lu L, Bagheri M, Lu Z, Summers R. ChestX-ray8: Hospital-scale Chest X-ray database and benchmarks on weakly-supervised classification and localization of common thorax diseases. IEEE Conference on Computer Vision and Pattern Recognition (CVPR). 2017, 2097-2106.
Acknowledgments
This work was supported by the Intramural Research Programs of the National Institutes of Health, National Library of Medicine and Clinical Center.
We are grateful to the authors of NegEx, MetaMap, Stanford CoreNLP, Bllip parser, and CheXpert labeler for making their software tools publicly available.
We thank Dr. Alexis Allot for the helpful discussion.
Disclaimer
This tool shows the results of research conducted in the Computational Biology Branch, NCBI. The information produced on this website is not intended for direct diagnostic use or medical decision-making without review and oversight by a clinical professional. Individuals should not change their health behavior solely on the basis of information produced on this website. NIH does not independently verify the validity or utility of the information produced by this tool. If you have questions about the information produced on this website, please see a health care professional. More information about NCBI's disclaimer policy is available.