Unlimiformer
This is the official implementation of the paper:
Amanda Bertsch, Uri Alon, Graham Neubig, and Matthew R. Gormley:
Unlimiformer: Long-Range Transformers with Unlimited Length Input
Unlimiformer is a method for augmenting pretrained encoder-decoder models with retrieval-based attention, without changing the mathematical definition of attention.
This allows the use of unlimited length inputs with any pretrained encoder-decoder!
See also our Tweet.
Unlimiformer can be used to improve the performance of an already-trained model. For best results, the model can be trained with Unlimiformer training.
If you have any questions on this work, please open a GitHub issue or email the authors at [email protected], [email protected]
August 2023 - Unlimiformer now supports Llama-2 (and all its derivatives)!
To prompt Llama-2 with extremely long inputs, for example, the content of an entire book, use:
python src/run_generation.py --model_type llama --model_name_or_path meta-llama/Llama-2-13b-chat-hf \
--prefix "<<SYS>>\n You are a helpful assistant. Answer with detailed responses according to the entire instruction or question. \n<</SYS>>\n\n [INST] Summarize the following book: " \
--prompt example_inputs/harry_potter_full.txt \
--suffix " [/INST]" --test_unlimiformer --fp16 --length 200 --layer_begin 16 \
--index_devices 1 --datastore_device 1
- The final prompt will be a concatenation of the content of the flags:
--prefix
,--prompt
,--suffix
. - The flag
--prompt
may contain either a path to a text file (e.g.,example_inputs/harry_potter_full.txt
) or the concrete prompt string. - The flag
--test_unlimiformer
is required to enable Unlimiformer. - The flag
--length
determines the desired output length. - The flag
--layer_begin
determines the layer from which Unlimiformer will start to be applied. For example, if we set--layer_begin 20
, the first 20 layers of the model will perform the standard attention over the lastcontext_window_size
tokens of the prompt as usual, and the 21st layer and above will attend to the entire long input. From our initial experiments, the value of--layer_begin
should be more than half of the total number of layers in the model, and tuning it dramatically changes the quality of the output. - The flags:
--datastore_device N
and--index_devices N1 N2 N3 ...
specify on which GPUs to store Unlimiformer's datastore and index (the base model will be stored on GPU #0). - Add the flag
--stream_output
to make the generated tokens appear one by one as they are generated.
Getting Started
General Instructions
Copy the files from src
into your source code folder.
You'll need to set values for the Unlimiformer-specific arguments outlined in usage.py
- you can add these arguments wherever you usually process hyperparameters. To use the model, you must set test_unlimiformer=True
. For datastore usage, the model must be in evaluation model (e.g. call model.eval()
before inference).
inference-example.py
outlines a minimal example for running a sequence through an Unlimiformer model, using the default arguments.
run.py
is an example of a full training setup that integrates Unlimiformer, adopted from SLED. See full command lines below.
Reproducing the Experiments from the Paper - Command Lines
To run a standard finetuning + evaluation of BART-base on the GovReport dataset (as examples), use:
python src/run.py \
src/configs/model/bart_base_sled.json
src/configs/training/base_training_args.json \
src/configs/data/gov_report.json \
--output_dir output_train_bart_base_local/ \
--learning_rate 1e-5 \
--model_name_or_path facebook/bart-base \
--max_source_length 1024 \
--eval_max_source_length 1024 --do_eval=True \
--eval_steps 1000 --save_steps 1000 \
--per_device_eval_batch_size 1 --per_device_train_batch_size 2 \
--extra_metrics bertscore
- To use Unlimiformer at test/validation time, use also:
--test_unlimiformer --eval_max_source_length 999999
- To use Unlimiformer at training time (called "Retrieval training" in the paper), use:
--unlimiformer_training --max_source_length 16384
- Alternatively, to use the computationally cheaper "Random-encoded" at training time, use
--random_unlimiformer_training --max_source_length 16384
- To altenate between "retrieval training" and "random-encoded training", use both flags:
--unlimiformer_training --random_unlimiformer_training --max_source_length 16384
For additional flags and options, see usage.py
Recommended settings
To evaluate with Unlimiformer
At evaluation time, we recommend the default value for each setting.
To train with Unlimiformer
For an inexpensive method, we recommend training as usual and using Unlimiformer during early stopping. To do so, set knn=True
and leave all other values at default.
For best performance, there are 3 expensive settings for training. The best one varies by dataset.
- Set
random_unlimiformer_training=True
: this is the random-encoded training setting from the paper - Set
unlimiformer_training=True
: this is the retrieval training setting from the paper - Set
random_unlimiformer_training=True
ANDunlimiformer_training=True
: this is the alternating training setting from the paper
See Table 5 in the paper for a more detailed breakdown of relative training costs.
Tips for very large inputs
For training
- you may need to truncate your inputs at training time, e.g. to 8k or 16k tokens. You can use the full inputs at evaluation time
- you can also try splitting your inputs into 16k-token-chunks and training on each one as its own example
For evaluation (including early stopping)
- if you're consistently running out of CUDA memory, set
use_datastore=True
to use a Faiss datastore to store hidden states. - if you're still having issues, set
gpu_datastore=False
orgpu_index=False
, but note that this will degrade performance
Trained models
The following models from the paper are available on Hugging Face. Please note that you must add the Unlimiformer-specific files to your repository, and load these models with test_unlimiformer=True
. If you download these models from Hugging Face, they may not use Unlimiformer by default!
Table 3: low-cost training methods
Dataset | Method | Hugging Face link |
---|---|---|
GovReport | Baseline: BART-base | abertsch/bart-base-govreport |
GovReport | BART-base + Unlimiformer early stopping | abertsch/unlimiformer-bart-govreport-earlyk |
SummScreen | Baseline: BART-base | abertsch/bart-base-summscreen |
SummScreen | BART-base + Unlimiformer early stopping | abertsch/unlimiformer-bart-summscreen-earlyk |
Table 4: Long-range training methods
Dataset | Method | Hugging Face link |
---|---|---|
GovReport | BART + Unlimiformer (alternating training) | abertsch/unlimiformer-bart-govreport-alternating |
SummScreen | BART + Unlimiformer (retrieval training) | abertsch/unlimiformer-bart-summscreen-retrieval |
Table 5: BookSum
Dataset | Method | Hugging Face link |
---|---|---|
BookSum | Baseline: BART-base | abertsch/bart-base-booksum |
BookSum | BART-base + Unlimiformer early stopping | abertsch/unlimiformer-bart-booksum-earlyk |
Booksum | BART-base + Unlimiformer (random-encoding training) | abertsch/unlimiformer-bart-booksum-random-encoding |
Booksum | BART-base + Unlimiformer (alternating training) | abertsch/unlimiformer-bart-booksum-alternating |
Results
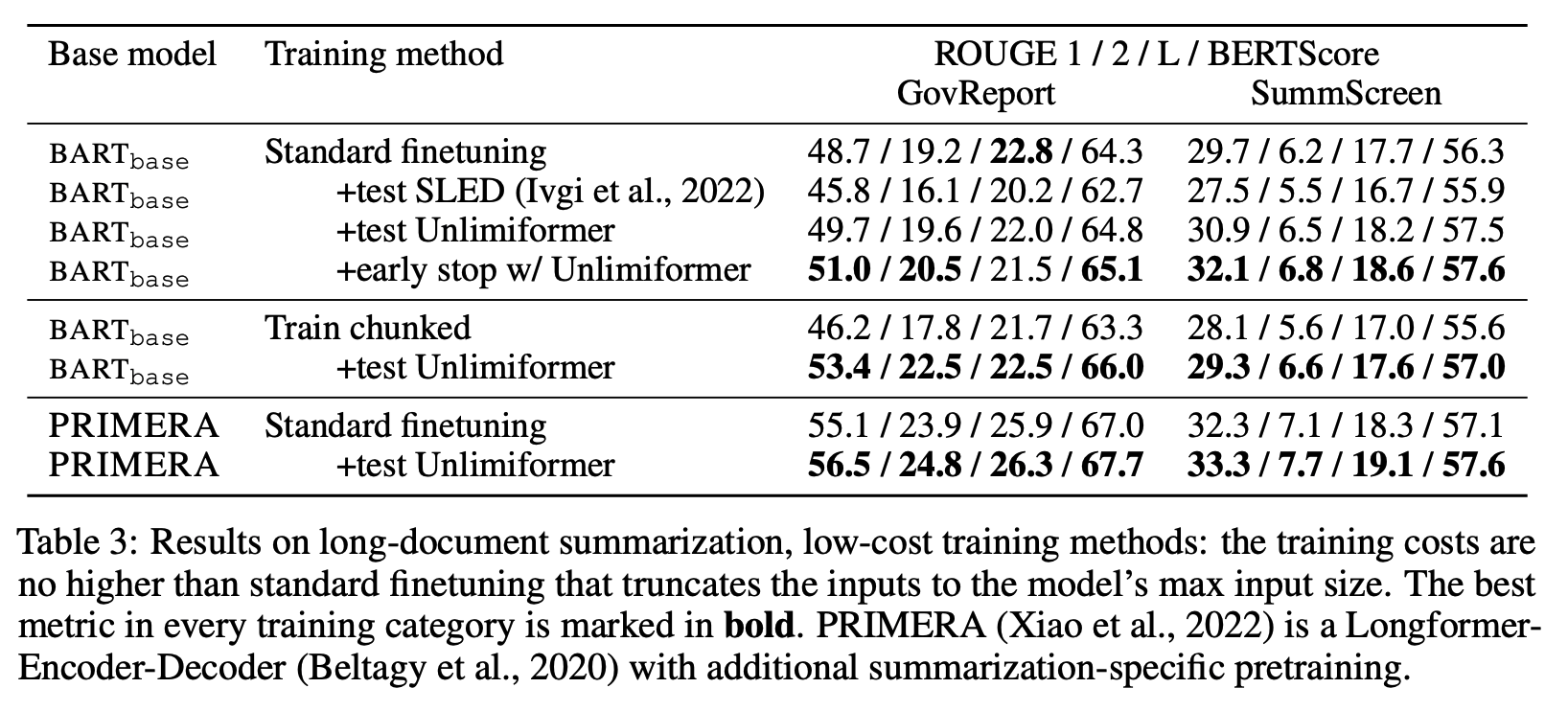
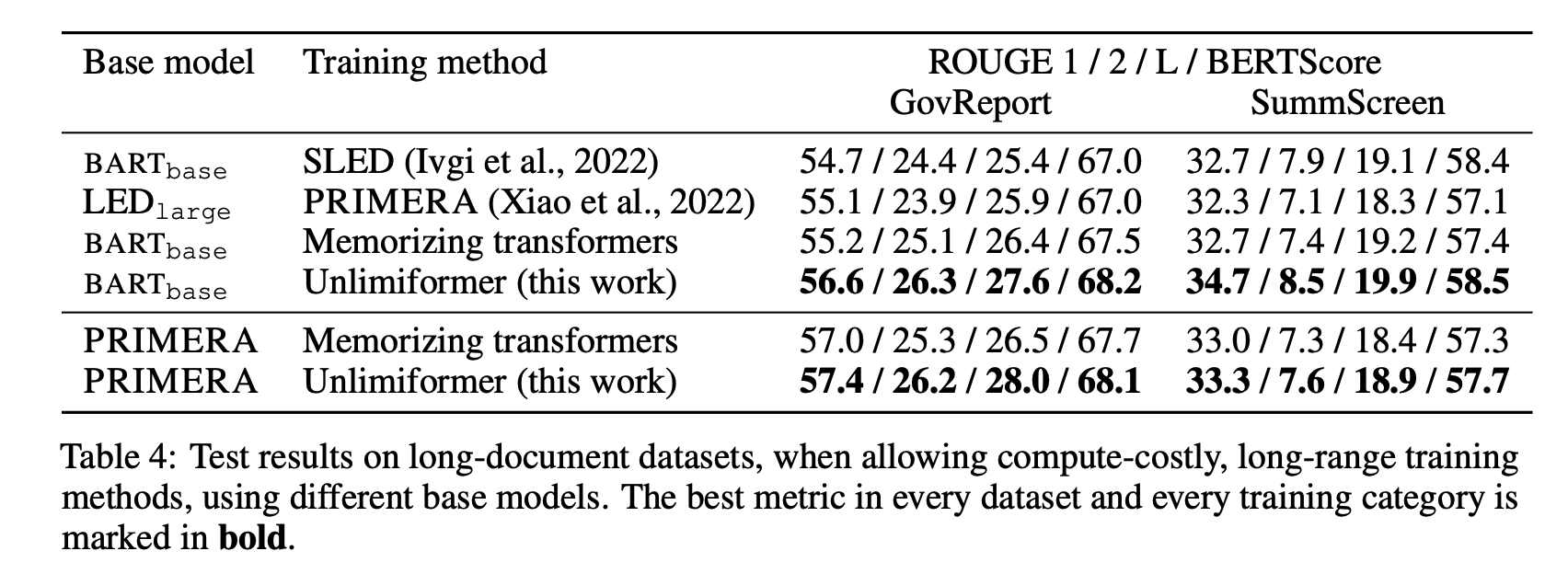
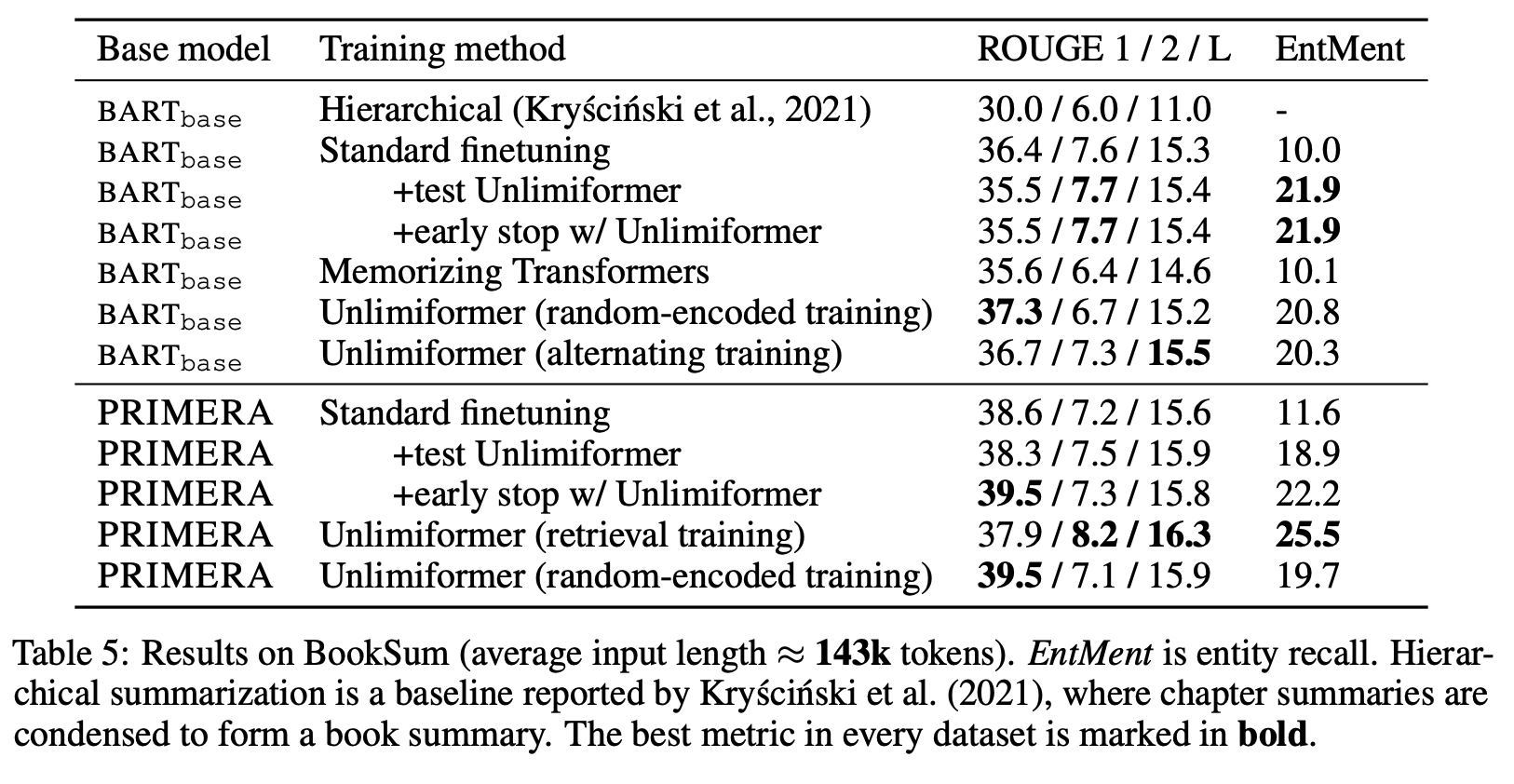
Citation
If you use our method or models, please cite our paper:
@article{bertsch2023unlimiformer,
title={Unlimiformer: Long-Range Transformers with Unlimited Length Input},
author={Bertsch, Amanda and Alon, Uri and Neubig, Graham and Gormley, Matthew R},
journal={arXiv preprint arXiv:2305.01625},
year={2023}
}